As societal ills proliferate, often beyond what we feel equipped to manage, so goes the potential for more people to fall between the cracks – of awareness, of acute intervention, of treatment. The opioid crisis is a national and particularly tragic case in point.
Facing the challenge head-on, researchers at Vanderbilt University Medical Center have teamed up with the Tennessee Department of Health (TDH) to work faster than the pace of the opioid epidemic. Their collaboration has led to two artificial intelligence (AI) models that could propel physicians, pharmacists and community-health leaders ahead to anticipate risk of fatal and nonfatal opioid-related overdoses in Tennessee.
“This AI project requires a complex approach, both in terms of assessing risk and strategies for intervention and optimizing resource allocation,” said Colin G. Walsh, M.D., an associate professor of biomedical informatics at Vanderbilt who is leading the project. “We want to shine a light wherever risk is to help those in need. To do that, we need the right data across our state and the right partnerships.”
“We want to make decisions before harm has occurred instead of reacting to what happened last month.”
Comprehensive Risk Assessment
In 2018, over 8,000 Tennessee residents overdosed on opioids, and 1,307 died. While Tennessee ranks 11th in the nation for drug overdose deaths, all states have suffered and have accepted the onus of forging mitigation strategies.
Most of these agencies and departments have focused their risk-assessing AI algorithms on Medicare claims, self-reported substance use patterns and demographics; some have included EMR data. Thus far, however, only a handful of states, including Tennessee, are looking at overdose using rich prescription drug monitoring program (PDMP) data. The approach taken by Vanderbilt and the TDH goes even further, combining PDMP output with information on those treated for opioid-related overdose from the Hospital Discharge Data System and Tennessee death certificate records.
“Combatting this evolving epidemic will demand innovative and novel approaches.”
The AI algorithm then amalgamates these data using an ensemble of factors that include zip code, insurance status, income, crowding, geographic access to substance use clinics and pharmacies and various public indices that measure vulnerability. Uniquely, the models were designed to predict point-of-prescription risk for overdose or death in the thirty days following each prescription.
“We want to make decisions before harm has occurred instead of reacting to what happened last month,” Walsh said.
Predicting Hotspots
Their reactive nature means that overdose prevention analyses direct attention to spots where tragedy has reached high levels based on deaths that have already occurred. This AI-enabled prescription-level risk strategy enables analysts to look at data in terms of regions, cities, neighborhoods and specific and recent time frames and determine where risk is trending.
“We’ve demonstrated our models’ ability to predict risk in the next thirty days at the prescription level,” Walsh said. “Now, we are testing risk aggregation to predict areas where we can say, ‘This might be the next hotspot for high overdose rates.’”
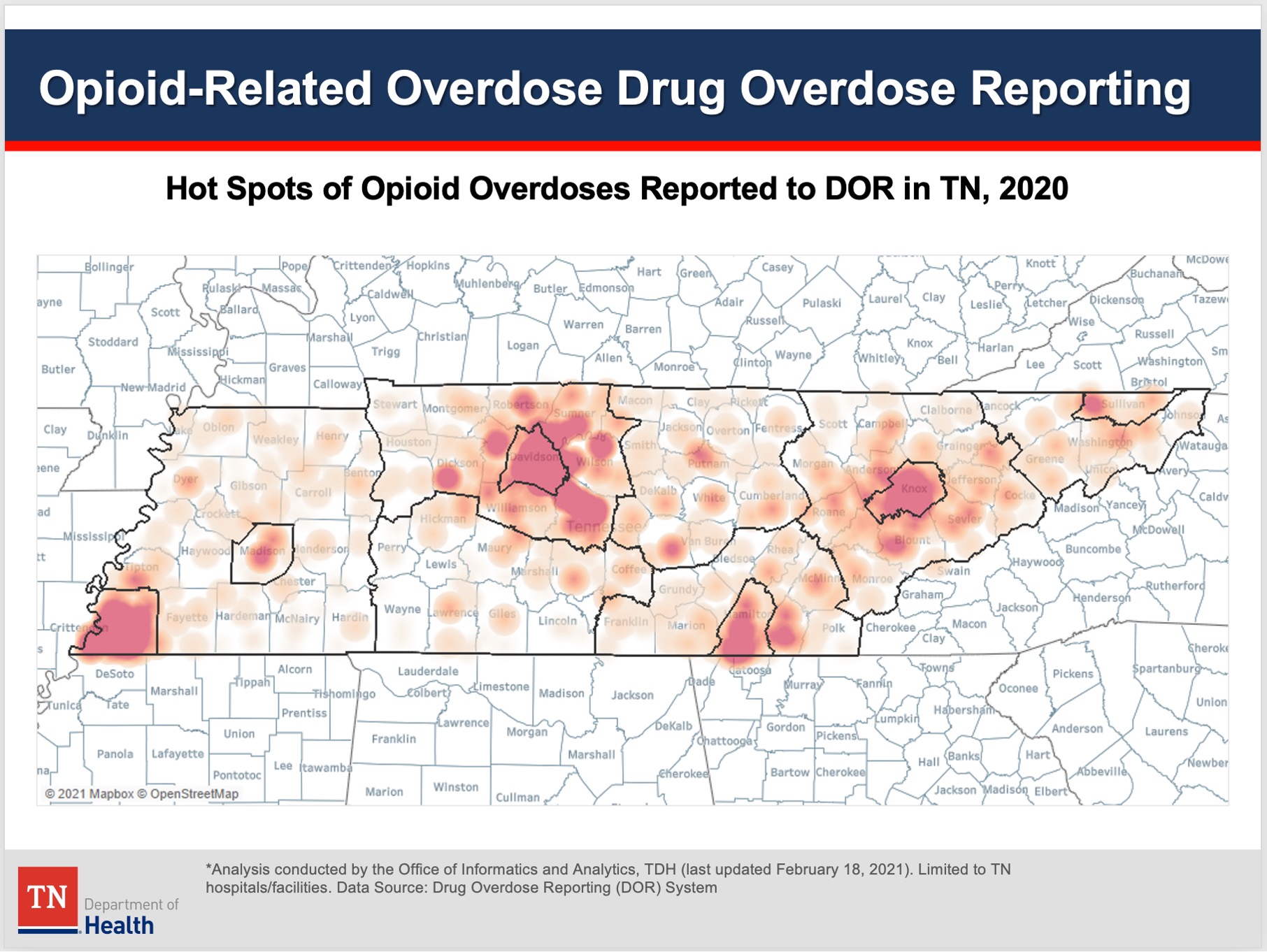
Studies testing how well the models predict counties and regions at the highest risk and in need of public health resource allocation are underway. “Our hope is that these models will allow Tennessee to proactively support the greatest at-risk communities with resources like clinics, staffing, public education and targeted interventions,” Walsh said.
Clinical Applications Still to Come
Walsh says that currently, both the fatal and nonfatal overdose models have a high number of false positives, making clinical application at the point-of-care inadvisable. However, the team is working on bringing these down to a level where, for example, a pharmacist might use the tool as a trigger to dig deeper into a prescribing decision.
“We’ve demonstrated our models’ ability to predict risk in the next thirty days at the prescription level.”
“Right now, the main actionability of these models focuses on community and geographic risk within the state of Tennessee, and potentially in other states,” Walsh said.
Risk Prediction Beyond Opioids
These models follow Walsh’s work with William Stead, M.D., McKesson Foundation Professor of biomedical informatics at Vanderbilt, on the successful testing of an AI tool for suicide prediction. This model is embedded in an EMR to supply real-world, point-of-care decision support interventions.
Looking ahead, Walsh is eyeing the challenge of using AI to predict the risks from the more deadly synthetic drug, fentanyl. The absence of prescription records for these illicit drugs limits both traceability and deployment of a predictive model, but Walsh says some researchers have successfully linked data on illicit drugs to other public health datasets.
“Combatting this evolving epidemic will demand innovative and novel approaches. It will also require committed partnerships like the one we have with the TDH to predict risk and prevent harm,” he said.